Summary
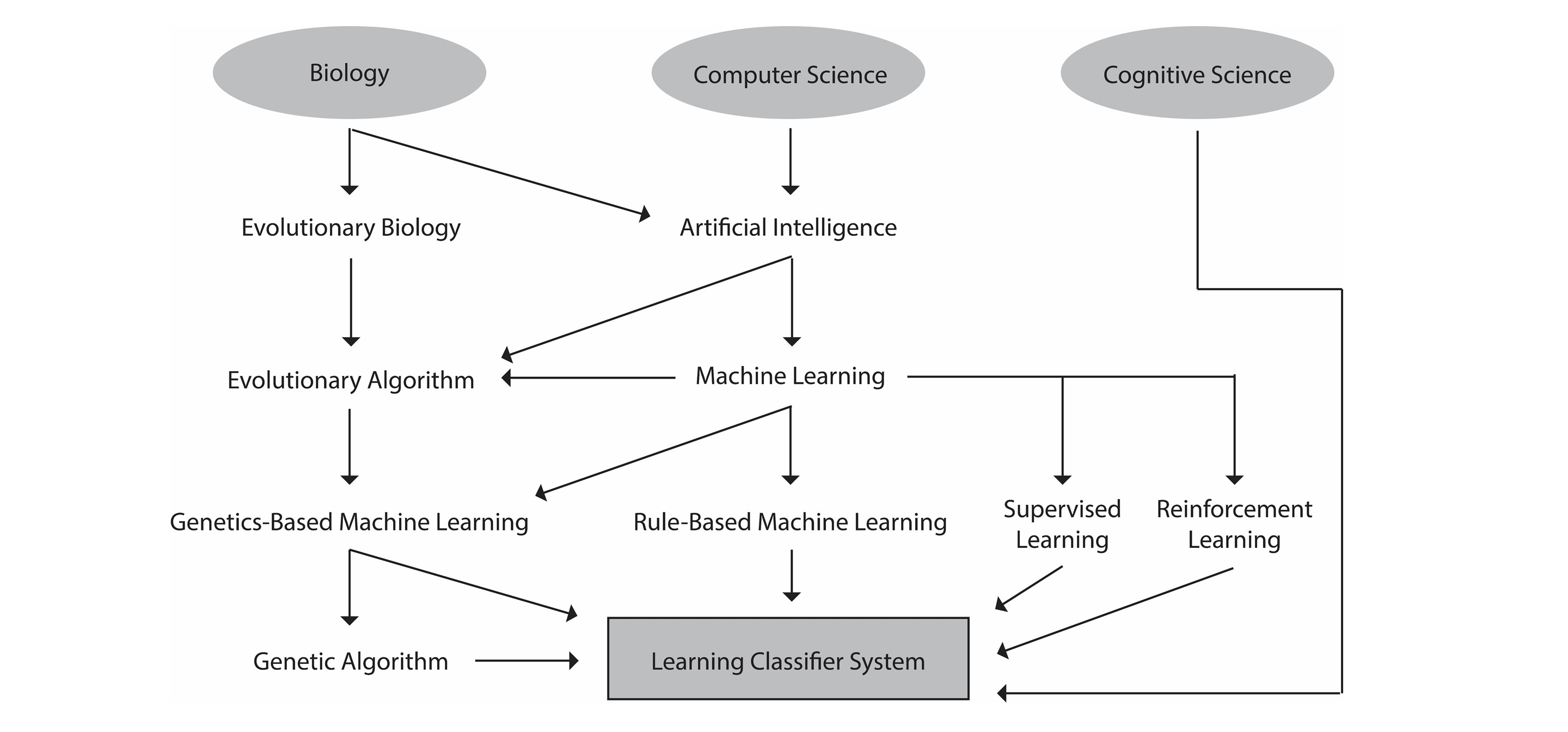
LCS field tree
The nature of Michigan-style LCSs impart them with a number of notable advantages: (1) they are model-free and thus do not make assumptions about the data (e.g. the association can be linear, epistatic, or heterogeneous; the number of predictive attributes; noisy or clean signal; balanced or imbalanced classes; how to handle missing data; or attributes being a mix of both discrete and continuous), (2) Individual rules are directly interpretable as IF:THEN expressions, (3) they are implicitly multi-objective, evolving rules toward maximal accuracy and generality (i.e. rule simplicity) to improve predictive performance, (4) on their own, they have themes in common with an ensemble learning system where individual rules or groups of rules propose different solutions within a collective rule population, (5) they are adaptive to changing dataset environments, such that parts of a solution can adapt without starting over from scratch, (6) by relying on evolutionary computation to explore the search space they offer a practical option when deterministic, exhaustive search is intractable, and (7) the algorithm is compartmentalized such that individual components can be updated or replaced to handle specialized problem domains. Key disadvantages of LCS include (1) the belief that they are somewhat more difficult to properly apply, (2) they lack a comparable theoretical understanding next to other, well-known machine learning strategies and are not guaranteed to converge on the optimal solution, (3) they are relatively computationally demanding and in certain problem domains can take longer to converge on a solution, and (4) most implementations to date have a relatively limited scalability.
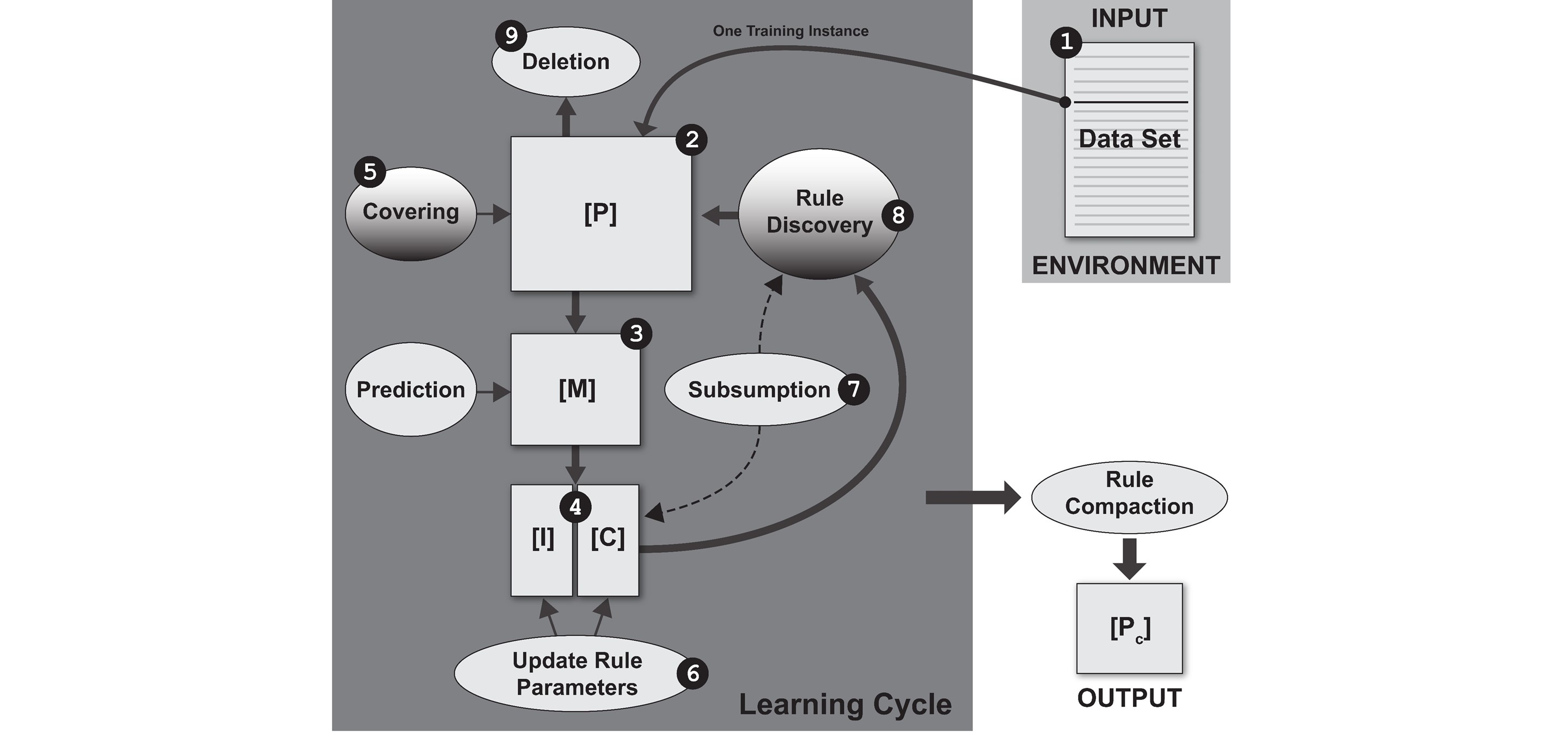
Schematic of a generic supervised learning classifier system.
Rule-Based Machine Learning Content
Review Papers
- Learning Classifier Systems: A Complete Introduction, Review, and Roadmap (2009)
- Learning Classifier Systems: A Brief Introduction (2004)
- What is a Learning Classifier System (2000)
Books
- Design and Analysis of Learning Classifier Systems: A Probabilistic Approach (2008)
- Learning Classifier Systems in Data Mining (2008)
- Rule-Based Evolutionary Online Learning Systems: A Principled Approach to LCS Analysis and Design (2006)
- Foundations of Learning Classifier Systems (2005)
- Applications of Learning Classifier System (2004)
- Strength or Accuracy: Credit Assignment in Learning Classifier Systems (2004)
Links
- Wikipedia – Learning Classifier System (This webpage and description is in great need of and update and new content. I will be revising this Wiki page at the request of the LCS community as soon as possible)
- GBML Central
- Clever Algorithms: Nature-Inspired Programming Recipes
Slideshows
- Introducing Rule-Based Machine Learning: Capturing Complexity (Urbanowicz 2016, Presented as GECCO Tutorial)
- Introducing Rule-Based Machine Learning: A Practical Guide (Urbanowicz and Browne 2015, Presented as GECCO Tutorial)
- Learning Classifier Systems Genetic Algorithms and Other Evolutionary Approaches (Lanzi 2014)
- Introduction to Learning Classifier Systems (Bacardit)